Freshness
Freshness means data relevancy and currency within the system. It ensures data is up-to-date and is available when necessary. It is important for processes requiring real-time data like stock trading platforms or real-time analytics in retail. Fresh data facilitates predictive analytics accuracy and decision-making. In the digital business market, stale data would cause errors during decision-making, leading to missed opportunities. Freshness checks alert teams about data update lapses or delays so that correct action can be taken.
Volume
It involves monitoring and keeping track of the amount of data produced and processed. Having volume checks helps identify inconsistencies that could result in issues during data creation or collection processes. For example, a sudden data volume drop could be due to a failure in the data ingestion pipeline. A sudden spike might be due to duplicate data or data collection errors. By monitoring data volume, businesses can keep a consistent data flow and use it for analytics and reporting.
Distribution
Analyzing data distribution is necessary to know the range and spared of data values. This pillar identifies dataset anomalies and outliers. For example, a sudden change in transaction value distributions in financial software could cause fraudulent activity. Data distribution observation maintains the predictive model’s integrity and ensures accurate analytics using representative data.
Schema
This pillar focuses on data structure and format and involves monitoring changes in data schema (addition, deletion, or modification of data fields). This is useful in cases where multiple systems consume data with specific structure requirements. Changes in the schema can cause incorrect data interpretation or break integrations. Constant monitoring detects issues early and maintains data consistency and reliability across the organization.
Lineage
Data lineage defines the data flow through the pipeline (origin to final destination). This pillar offers a clear view of data transformation and its flow across multiple systems. Lineage tracking is necessary to diagnose issues, understand their impact, and ensure accountability. It is important in the regulatory compliance process, as businesses need to prove the origin of the data and its transformation.
How Does Data Observability Help in Achieving BI Goals?
With the digital business environment advancements, the need to interpret data accurately is highly important. Data observability ensures that the data supporting BI tools is reliable, resulting in effective business decisions and strategies.

Data Accuracy and Reliability
Accurate data is crucial for effective BI. Businesses can use data observability tools to monitor data quality and set alerts for inconsistencies to improve the efficiency of BI insights and decision-making. It will help prevent costly mistakes caused by data errors.
Manage Issues Swiftly
Observability tools facilitate real-time issue identification, allowing businesses to take immediate action. This approach helps prevent minor problems from escalating and ensures BI tools operate on clean and accurate data. For instance, setting immediate alerts for data pipeline failures can save time and resources.
Risk Management
Implementing comprehensive data observability procedures enables businesses to anticipate and mitigate risks faster and more effectively. It gives a complete view of data health and market trends, allowing businesses to make informed decisions on risk management, a key aspect of BI.
Reporting and Compliance
It becomes easy to track data lineage with observability tools, making it feasible to report on data usage and transformation within the business processes. This is also important for compliance with data protection regulations. Detailed reporting also provides reliable insights from BI tools.
Improved BI Performance
Observability tools maintain the data quality and ensure timely delivery for BI operations. It leads to better performance of BI tools as they provide faster and more accurate insights based on updated data.
Better Understanding of Customer Behavior
High-quality data helps businesses better understand their customer behavior and requirements. Observability tools accuracy of data used in analyzing customer preferences and trends, resulting in effective engagement strategies.
Top 5 Data Observability Tools
When choosing data observability tools, make sure to select ones that sync with BI-specific needs. Here are the top 5 data observability tools for 2024:
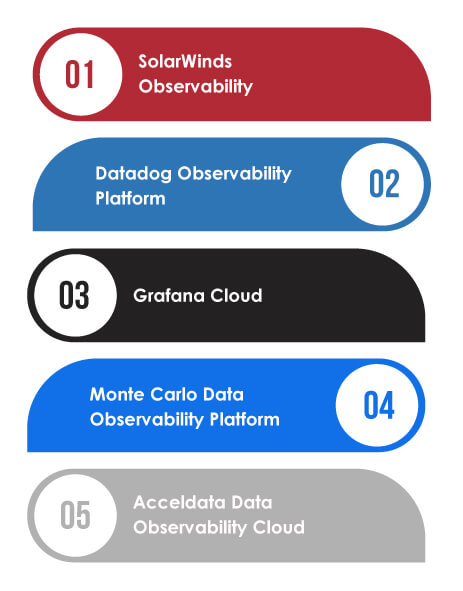
SolarWinds Observability
It provides a comprehensive and unified view of IT infrastructure, which includes data from logs, traces, metrics, etc. This tool effectively manages and monitors distributed environments, and it supports open-source frameworks and third-party integrations.
Datadog Observability Platform
Datadog is known for handling complex technology stack as it can easily integrate with over 700 technologies (such as AWS, Kubernetes, Slack, etc.). This observability platform combines traces, logs, and metrics for E2E visibility. It has an AI-powered anomaly detection feature that monitors various technologies.
Grafana Cloud
This tool focuses on observability cost management. It facilitates real-time monitoring and customization of dashboards, enabling effective management of costs.
Monte Carlo Data Observability Platform
This observability platform automates root cause analysis and offers a detailed view of data (including data lakes, warehouses, BI tools, etc.). The Monte Carlo data observability platform is easy to set up and seamlessly integrates with data stacks.
Acceldata Data Observability Cloud
Acceldata is a multi-dimensional platform focusing on data reliability, data pipeline performance optimization, and reduction of inefficiencies. The product stack easily integrates with data stacks like ETL tools and orchestration pipelines.
Summary
Data observability is an important asset for achieving business intelligence goals in the digital business environment. It ensures that data used for decision-making is accurate, reliable, and of high quality. It involves a holistic approach to understanding data health within the system and addressing critical issues like data integrity and downtime. Observability impacts various business aspects, from enhancing customer insights to ensuring data-driven compliance. However, one must partner with a professional data observability service provider like TestingXperts to ensure its effective integration with BI operations.
Why Partner with Tx for Data Observability Services?
Trustworthy data is vital for today’s enterprises using analytics to identify opportunities and feed AI/ML models to automate decision-making. TestingXperts offers comprehensive data observability solutions to ensure your data is reliable and you get the following benefits:
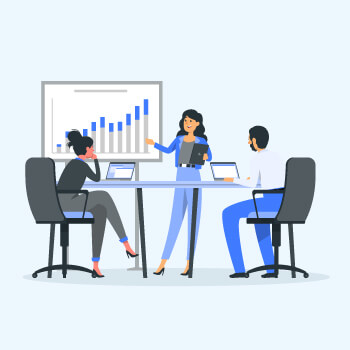
• Eliminate risks associated with inaccurate analytics by detecting and addressing data errors before they disrupt your business and lead to costly downstream problems.
• Use effective solutions to trace the root cause of an issue, along with remediation options to resolve issues efficiently and quickly.
• Proactively identify and eliminate issues to minimize the cost associated with adverse data events.
• Empower your data engineers and other stakeholders with a thorough understanding of your data to support your digital transformation initiatives.
• Implement AI and RPA-driven quality engineering for efficient and effective automation.
To know more, contact our QA experts now.